The Evolution of Personalized Marketing
The days of one-size-fits-all marketing are firmly behind us. Today’s consumers expect experiences tailored to their preferences, behaviors, and needs—and AI-powered personalization has emerged as the most effective way to deliver these customized interactions at scale.
AI-powered personalization uses machine learning algorithms to analyze customer data, identify patterns, and automatically deliver tailored content, product recommendations, or experiences to each individual. Unlike rules-based personalization that relies on manual segmentation, AI systems continuously learn and adapt based on new data, creating increasingly relevant experiences over time.
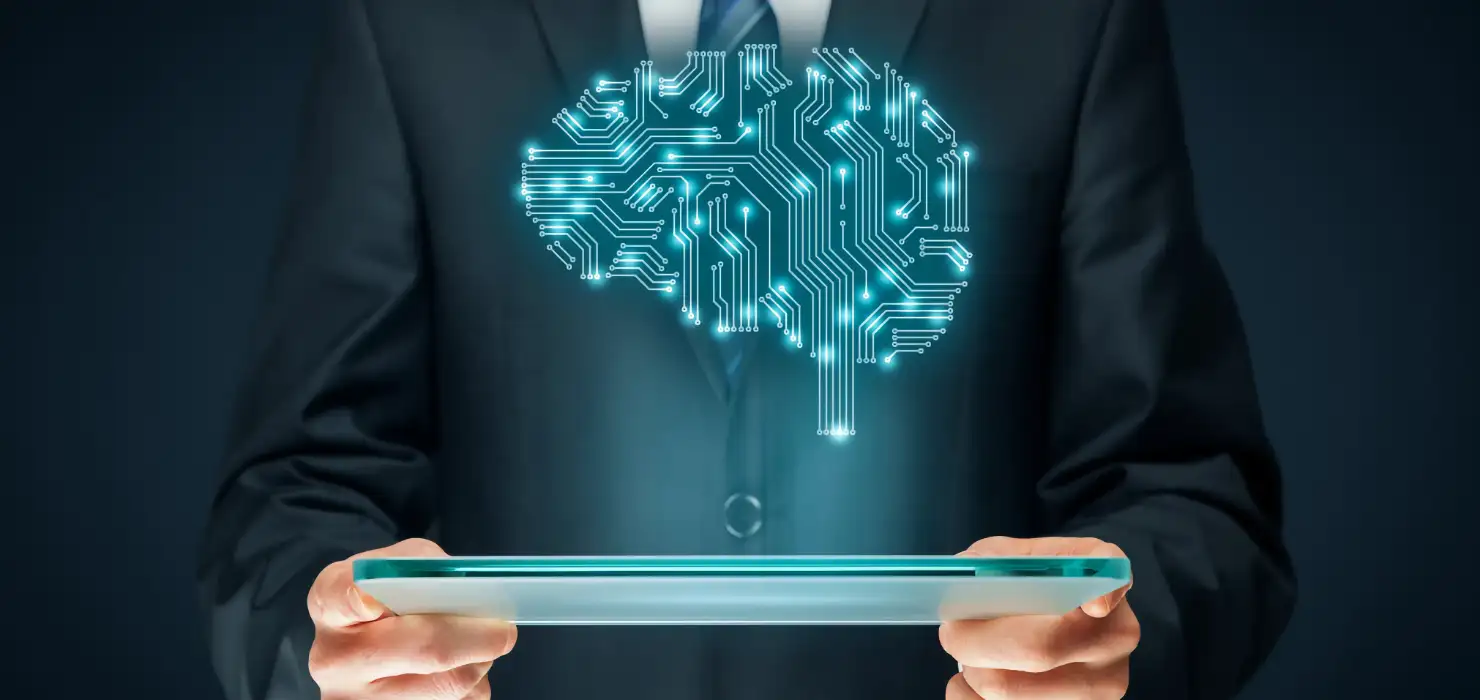
The impact of well-implemented AI personalization is substantial. According to a comprehensive study by McKinsey, companies that excel at personalization generate 40% more revenue from these activities compared to average performers. Yet many businesses struggle with implementation, often believing that effective AI personalization requires enterprise-level resources and technical expertise.
The truth is that AI-powered personalization exists on a spectrum, with options available for organizations of every size and technical capability. This article explores practical implementation approaches from basic to advanced and outlines privacy-compliant methods to deliver personalized experiences that respect user data.
Implementation Options from Basic to Advanced
Entry-Level AI Personalization Solutions
Contrary to popular belief, getting started with AI personalization doesn’t require a data science team or massive technology investments. Several approachable options allow businesses to implement basic personalization with minimal resources:
Plug-and-play personalization tools:
- Email marketing platforms with built-in AI capabilities (Mailchimp, Campaign Monitor, Klaviyo) that automatically optimize send times, subject lines, and content based on individual engagement patterns
- Website personalization widgets (RightMessage, Optimizely) that display different content based on visitor attributes or behaviors without requiring custom development
- E-commerce recommendation engines (Nosto, Clerk.io) that integrate with popular platforms to deliver product recommendations based on browsing and purchase history
These solutions typically require only basic configuration rather than technical implementation, making them accessible even for small marketing teams.
Low-code implementation approaches:
- Personalized retargeting using platforms like Facebook Custom Audiences or Google’s Dynamic Remarketing to show ads based on specific product interactions
- Content recommendation systems that suggest relevant articles or resources based on previous consumption patterns
- Behavioral email triggers that send automated messages based on specific user actions or inactions
The key to success with entry-level personalization is focusing on high-impact use cases rather than attempting to personalize every customer touchpoint. Begin with areas where personalization most directly influences conversions—typically product recommendations, abandoned cart recovery, or content suggestions.
Best practices for basic implementation:
- Start with a single channel where you have the most customer data
- Focus on one personalization use case before expanding
- Use pre-built templates and workflows rather than custom solutions
- Establish clear baseline metrics to measure impact
Even basic AI personalization significantly outperforms generic marketing approaches, making it a worthwhile investment for businesses just beginning their personalization journey.
Intermediate and Advanced Implementation Approaches
As organizations grow more comfortable with AI personalization and collect more customer data, more sophisticated approaches become feasible:
Mid-level personalization capabilities:
- Cross-channel personalization orchestration that maintains consistent experiences across email, website, mobile, and ads
- Predictive personalization that anticipates customer needs based on behavioral patterns rather than just reacting to them
- Dynamic content generation that automatically creates variations of marketing assets tailored to different audience segments
- Personalized pricing and promotional strategies that optimize offers based on individual price sensitivity
These approaches typically involve dedicated personalization platforms (e.g., Dynamic Yield, Evergage) or custom integration between existing marketing technologies using APIs and customer data platforms.
Advanced AI personalization systems:
- Real-time personalization engines that instantly adapt experiences based on current session behavior
- Natural language generation for creating completely individualized content at scale
- Visual recognition systems for personalized shopping experiences based on style preferences
- Predictive lifetime value optimization that personalizes experiences based on projected future value
- Multivariate personalization that simultaneously tests multiple personalization approaches to identify optimal combinations
Advanced implementations generally require dedicated technical resources, either internal teams or specialized agency partners, and significantly more sophisticated data infrastructure.
Implementation considerations as you advance:
- Data integration becomes increasingly important—invest in unifying customer data across sources
- Governance and testing frameworks help prevent personalization from creating inconsistent brand experiences
- Regular algorithm training and refinement produce progressively better results
- Measurement must evolve beyond simple engagement metrics to assess business impact
While advanced implementations require greater investment, they typically deliver proportionally higher returns by creating truly individualized experiences that significantly increase customer lifetime value.
Privacy-Compliant Approaches to AI Personalization
Balancing Personalization and Privacy
The personalization paradox is real: consumers simultaneously want tailored experiences and express concerns about how their data is used. Implementing AI personalization in a privacy-compliant way isn’t just about regulatory compliance—it’s about building trust with your audience.
Core privacy principles for personalization:
- Transparency about data collection and usage
- Consent that gives users real choice about personalization
- Control that allows users to manage their preferences
- Data minimization that collects only what’s necessary
- Purpose limitation that uses data only for its stated purpose
These principles align with global privacy regulations like GDPR, CCPA, and emerging standards, while also addressing consumer expectations about data usage.
Privacy-by-design personalization approaches:
- Zero-party data personalization: Prioritize personalization based on information customers proactively share (preferences, interests, goals) rather than inferred data. This approach both respects privacy and often delivers more accurate personalization since it’s based on explicit rather than implicit signals.
- Contextual personalization: Personalize based on current session behavior rather than persistent profiles. This approach tailors experiences based on immediate intent signals without requiring extensive data history.
- Anonymized pattern recognition: Use anonymized or aggregated data to identify behavior patterns that inform personalization without tracking specific individuals. This technique is particularly valuable for content recommendation and product suggestion systems.
- Edge computing personalization: Process personalization algorithms on the user’s device rather than sending data to central servers. This emerging approach keeps sensitive data on the user’s side while still delivering tailored experiences.
- Differential privacy techniques: Apply mathematical approaches that add “noise” to datasets, preserving individual privacy while allowing accurate pattern analysis for personalization algorithms.
Technical safeguards for privacy-compliant personalization:
- Implement proper consent management platforms that enforce user preferences
- Adopt data minimization practices that only collect information with clear personalization value
- Create data retention policies that automatically purge unnecessary information
- Deploy strong data security measures that protect personalization data
- Establish regular privacy audits of personalization systems
By implementing these privacy-centered approaches, businesses can deliver highly personalized experiences while respecting user data rights—creating sustainable personalization programs that build rather than erode trust.
Implementation Blueprint: Starting Your AI Personalization Journey
For businesses ready to implement AI-powered personalization, here’s a practical blueprint that requires minimal initial resources:
- Assessment and Planning (2-3 weeks)
- Audit existing customer data sources and quality
- Identify high-impact personalization opportunities based on customer journey analysis
- Select an initial channel and use case with clear success metrics
- Establish privacy guidelines and compliance requirements
- Start Simple with Integrated Solutions (1-2 months)
- Implement a personalization solution that integrates with your existing marketing stack
- Begin with recommendation-based personalization (products, content) using pre-built algorithms
- Focus on a single high-traffic customer touchpoint
- Collect explicit preferences through preference centers or onboarding processes
- Ensure proper consent management and privacy controls
- Measure, Learn, and Expand (Ongoing)
- Establish A/B testing comparing personalized vs. non-personalized experiences
- Analyze results to identify which personalization elements drive the most impact
- Gradually expand to additional channels while maintaining consistent experiences
- Increase sophistication by incorporating more data sources and advanced algorithms
- Regularly audit for privacy compliance and effectiveness
- Build Your Personalization Ecosystem (3-6 months)
- Create a unified customer data platform to power more sophisticated personalization
- Implement cross-channel personalization capabilities
- Develop personalization governance to ensure brand consistency
- Establish more advanced measurement frameworks linking personalization to business outcomes
This phased approach allows organizations to realize immediate benefits from AI personalization while building toward more advanced capabilities over time—all while maintaining privacy compliance and delivering value at each stage.
Personalization as a Competitive Advantage
AI-powered personalization represents one of the most significant opportunities for businesses to differentiate themselves in increasingly crowded markets. By delivering experiences that feel individually crafted rather than mass-produced, organizations create stronger emotional connections with customers and drive meaningful business results.
The most successful personalization initiatives balance technological capabilities with human insight, using AI to scale personalization while maintaining the authentic feel that customers crave. And they do so while respecting privacy preferences and building trust through transparent data practices.
Whether you’re just beginning to explore basic personalization techniques or ready to implement advanced AI-driven experiences, the time to start is now. Each step toward more relevant, personalized customer interactions creates compounding benefits as your systems learn and improve over time.
Ready to develop a personalized approach to AI-powered marketing that fits your business goals and resource constraints? Contact our team at CargoLabs for a personalization readiness assessment and customized implementation recommendations.